The role of machine learning in stock market predictions
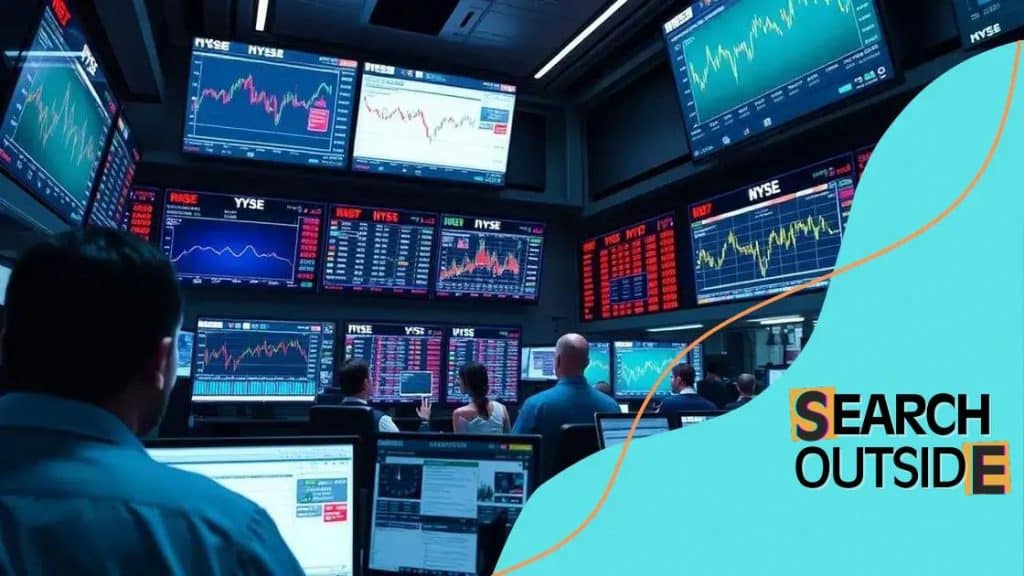
Anúncios
The role of machine learning in stock market predictions involves utilizing algorithms to analyze data, identify trends, and enhance decision-making, leading to more accurate forecasting and better investment strategies.
The role of machine learning in stock market predictions is more crucial than ever. With algorithms analyzing vast amounts of data, investors are discovering insights that could change their approach to trading. Ever wondered how a simple algorithm could transform your investment strategy?
Anúncios
Understanding machine learning in finance
Understanding machine learning in finance is vital to grasping how these systems can enhance decision-making. By using complex algorithms, financial institutions analyze vast datasets rapidly, leading to better predictions and strategies.
What is Machine Learning?
Machine learning is a subset of artificial intelligence that enables systems to learn from data. In finance, it helps in predicting market trends, managing risk, and even automating trading. This technology is not just for tech companies; banks and investment firms leverage it for efficiency.
Key Applications in Finance
There are several key applications of machine learning within the finance industry, including:
Anúncios
- Fraud detection: Identifying unusual patterns in transactions.
- Risk assessment: Evaluating the likelihood of default on loans.
- Algorithmic trading: Making quick buy/sell decisions based on data analysis.
- Portfolio management: Optimizing asset mixes for better returns.
The ability to process data in real-time sets machine learning apart, granting firms insights that were previously unimaginable. As technology advances, the importance of these algorithms grows exponentially.
How Machine Learning Changes Finance
Machine learning has transformed the finance landscape. Traditional methods relied on historical data analysis, which could be slow and often missed critical insights. With machine learning, predictions can be made using thousands of variables and real-time data, offering a more dynamic approach.
Moreover, it allows for automation in areas like customer service through chatbots, improving client interaction without human intervention. As machine learning continues to evolve, its integration into finance will deepen, reshaping the industry.
Key algorithms used for stock predictions
Key algorithms used for stock predictions are crucial for identifying patterns and trends in market data. These algorithms analyze historical and real-time data to forecast future stock prices, helping investors make informed decisions.
Linear Regression
One of the simplest techniques, linear regression predicts stock prices by establishing a relationship between the stock and one or more independent variables. This method assumes a linear relationship, making it easy to interpret but often limited in its accuracy.
Decision Trees
Decision trees break down data into smaller subsets while developing an associated decision tree incrementally. This approach helps understand which factors most influence stock prices. It can handle both numerical and categorical data effectively, offering clear visualizations.
Neural Networks
Inspired by the human brain, neural networks consist of interconnected nodes (neurons) that analyze complex datasets. They excel in identifying intricate patterns but require large amounts of data and computational power.
- Can model non-linear relationships effectively.
- Adapt well to changing market conditions.
- Useful for high-frequency trading.
Random Forest is another popular algorithm that combines multiple decision trees to improve prediction accuracy. By aggregating results from various trees, this method reduces overfitting and increases reliability.
These algorithms represent a tiny fraction of the many techniques available in stock market predictions. The continual evolution of algorithms alongside advanced data analytics is crucial for enhancing prediction accuracy.
Challenges faced in market predictions
Challenges faced in market predictions are significant and can impact the effectiveness of machine learning models. Despite advances in technology, several hurdles persist in accurately forecasting stock prices.
Data Quality
One of the primary challenges is the quality of data used for predictions. Inconsistent, incomplete, or erroneous data can lead to misleading results. Accurate data collection is crucial for creating reliable models.
Market Volatility
Market behavior can change rapidly due to economic events, political instability, or sudden market reactions. This volatility makes it difficult for models to adapt, as conditions affecting stock prices can fluctuate unexpectedly.
- Changes in regulations can impact the market.
- Natural disasters may cause abrupt market shifts.
- Investor sentiment and psychology greatly influence stock movements.
Another challenge is understanding the complex interactions between various market factors. Stock prices are influenced by numerous variables, and isolating these can be daunting. The interplay of market sentiment, economic indicators, and global events often introduces uncertainty.
Overfitting
Overfitting occurs when a model is too complex, capturing noise in the training data instead of the actual trend. This can result in poor performance when applied to new data. Striking the right balance between model complexity and generalizability is essential.
Additionally, the lack of transparency in many models, especially with deep learning techniques, can make it hard to understand how predictions are made. When stakeholders cannot interpret the results, trust in the model diminishes.
Real-life applications of machine learning
Real-life applications of machine learning are transforming various industries, including finance. By harnessing these technologies, businesses can make more accurate predictions and improve decision-making processes.
Predictive Analytics
One notable application is in predictive analytics, where machine learning models analyze historical data to forecast future outcomes. In finance, this is crucial for assessing risk and forecasting stock movements.
Algorithmic Trading
Machine learning algorithms are extensively used in algorithmic trading. These algorithms process vast amounts of data, allowing traders to execute transactions within milliseconds. By analyzing market conditions, they can identify opportunities and optimize strategies.
- Automated trading strategies increase efficiency.
- Algorithms adapt to changing market conditions.
- Speed enhances the potential for profit.
Credit scoring is another significant application. Machine learning models evaluate the creditworthiness of individuals by analyzing multiple factors, such as payment history and income levels. This leads to quicker decisions, benefiting both lenders and borrowers.
Fraud Detection
Detecting fraud is critical for financial institutions. Machine learning systems analyze transaction patterns to identify unusual behaviors. For instance, they can flag transactions that deviate from a user’s usual spending habits. This proactive approach helps reduce losses from fraudulent activities.
Customer service also benefits from machine learning through chatbots that provide instant support. These AI-driven tools can answer queries, suggest products, and even assist in completing transactions. By automating customer interactions, companies improve efficiency and user experience.
Future trends in stock market analytics
Future trends in stock market analytics are set to revolutionize how investors approach trading. As technology advances, these trends will significantly impact the financial landscape.
Increased Use of Artificial Intelligence
One major trend is the increasing use of artificial intelligence in stock market analysis. AI can process vast amounts of data faster than any human, allowing for more accurate predictions. Machine learning algorithms will become more sophisticated, adapting to new data and market changes in real-time.
Big Data Integration
Another key trend is the integration of big data into analytics. Financial analysts will use data from various sources, including social media, news articles, and market reports. This comprehensive approach will give investors a broader view of market sentiment, driving better investment decisions.
- Sentiment analysis tools will gauge public opinion from social media.
- News analytics will track how news impacts stock prices.
- Alternative data sources will provide insights beyond traditional metrics.
Moreover, the adoption of cloud computing will allow for more scalable and cost-effective analytics solutions. Investors can access real-time data and models from anywhere, enabling quicker decision-making.
Enhanced Visualization Tools
As stock market analysis evolves, so will visualization tools. Advanced graphics will help investors quickly interpret complex data. Tools will be more user-friendly, allowing even novice investors to make sense of intricate market dynamics.
Finally, regulatory changes will also shape the future of analytics. As governments adapt to new technologies, they will likely impose regulations that affect how data is collected and used, ensuring transparency while promoting innovation.
In conclusion, the future of stock market analytics is bright, driven by advancements in technology and data integration. With the increasing use of artificial intelligence and machine learning, investors will be able to make more accurate predictions. The integration of big data will provide deeper insights into market dynamics, while enhanced visualization tools will make it easier for everyone to understand complex information. As we move forward, adapting to regulatory changes will ensure that innovations continue to thrive in a responsible manner.
FAQ – Frequently Asked Questions about Machine Learning in Stock Market Predictions
How does machine learning improve stock market predictions?
Machine learning analyzes vast amounts of data quickly, identifying complex patterns and trends that humans may miss, leading to more accurate predictions.
What are some common algorithms used for stock predictions?
Common algorithms include linear regression, decision trees, and neural networks, each offering unique advantages for analyzing market data.
What challenges do investors face using machine learning?
Investors face challenges like data quality, market volatility, and overfitting, which can affect the reliability of predictions.
What are the future trends in stock market analytics?
Future trends include increased use of artificial intelligence, integration of big data, enhanced visualization tools, and adapting to regulatory changes.